Key Insights
Optimization Techniques Enhance AI Model Outputs
Optimization techniques play a crucial role in refining AI model outputs, especially when dealing with real-world constraints. These methods allow for the incorporation of business rules, human insights, and additional factors that pure machine learning models might overlook. By applying optimization on top of ML predictions, businesses can create more practical and effective solutions. This approach is particularly valuable in fields like advertising, marketing, and inventory management, where multiple factors influence decision-making. Optimization helps bridge the gap between theoretical predictions and real-world applications, ensuring that AI solutions align with business goals and constraints.
Democratization of Financial Information Through Language Models
Language models are poised to revolutionize the financial industry by making complex information more accessible to the average person. As these models improve, they will be able to understand and explain financial concepts, products, and services in simpler terms. This democratization of financial knowledge will empower individuals to make more informed decisions about their finances. For financial institutions, this shift means adapting their services to cater to a more educated customer base. The challenge lies in leveraging these language models to provide value while maintaining regulatory compliance and ensuring the accuracy of information presented to customers.
Solid AI Knowledge Creates Competitive Advantage in Industry
Building a deep understanding of AI tools and techniques is crucial for professionals looking to create a competitive advantage in the industry. Simply knowing how to call an API or use pre-built models is not enough in today’s competitive landscape. To truly stand out, individuals must comprehend the underlying mechanisms of AI systems, be able to customize them for specific needs and understand their limitations. This in-depth knowledge allows professionals to innovate, solve complex problems, and add unique value to their organizations. Continuous learning and hands-on experience with AI technologies are essential for maintaining this competitive edge in a rapidly evolving field.
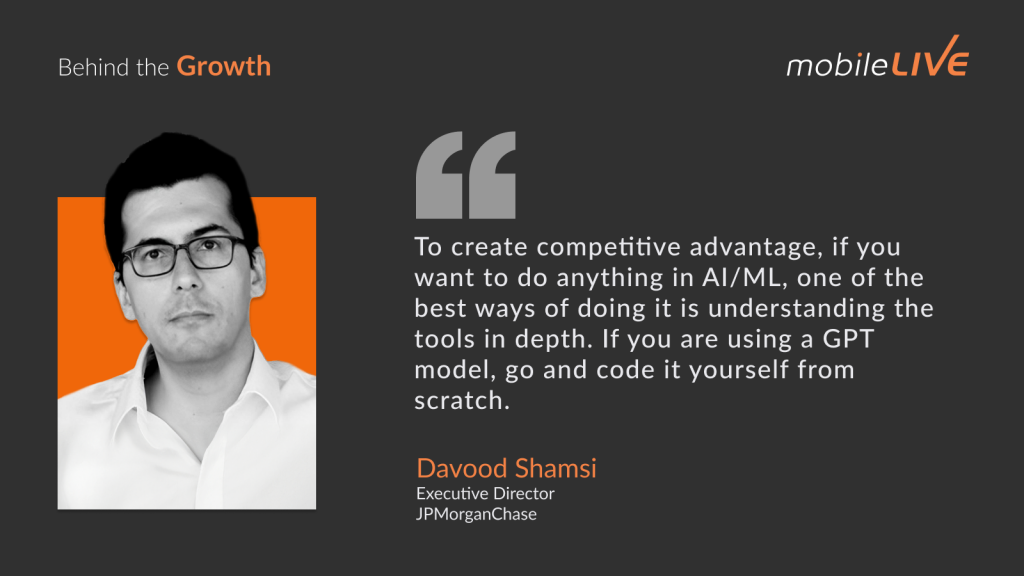
Episode Highlights
From Academia to Industry: A Unique Journey in AI
Davood Shamsi shares his unconventional entry into the field of AI and machine learning, starting with his pursuit to avoid military service in Iran. This initial motivation led him to study statistics and statistical modeling, eventually culminating in a Ph.D. in applied math and statistics. The transition from academia to industry presented new challenges, particularly in learning to work with diverse teams and focusing on practical applications rather than theoretical research. This shift allowed Davood to see the real-world impact of his work, especially in the advertising domain.
“I needed to learn how to transition from being the smartest person in the room to being able to work with the smartest people in the world. It’s a huge transition because you don’t need to be the smartest person when you’re starting or leading a project, especially a bigger project.”
Understanding Business Problems Before Applying AI Solutions
Davood emphasizes the importance of thoroughly understanding the business problem before proposing AI solutions. He suggests digging deep into the core issues, often uncovering better solutions than initially proposed. This approach involves educating business partners about AI capabilities while also learning about their specific needs and goals. By fostering this two-way relationship, AI teams can build trust and deliver more effective solutions that truly address the underlying business challenges.
“The interesting part is when we ask this question, sometimes our business partners start rethinking even their assumption about what they’re doing because we are trying to ask like, ‘What’s your goal?’ Sometimes they say, ‘You know what? I wanna improve this.’ I say, ‘Why? Why do you want to improve this? How does it help the business?’”
The Future of AI in Banking and Customer Service
Davood discusses the future of AI in banking, particularly focusing on customer service improvements. He explains how generative AI and language models can enhance understanding of customer needs without generating potentially risky responses. This approach allows for more efficient and accurate direction of customers to relevant information, ultimately improving the overall customer experience. The key is to use AI to comprehend user intent and match it with existing, verified information rather than generating new content.
“What you want to do, you want to use this technology to understand what exactly the user wants — which was impossible before. You could do it somehow, right? And then from there, now I know what you want. How can I use the information that is available and the text which is available to choose the best part of this text to show it to you.”
Preparing for the Future of AI and ML
In discussing how to prepare for a career in AI and ML, Davood emphasizes the importance of building a solid foundation in the core technologies. He advises aspiring professionals to go beyond simply using pre-built tools and APIs, encouraging them to understand the underlying mechanisms of AI systems. This deep knowledge allows for innovation and problem-solving in ways that create true competitive advantages. Additionally, Davood suggests that individuals should identify and leverage their unique strengths within the field, as different roles and specializations exist within the broad AI landscape.
“Building a solid knowledge about AI/ML in the sense that you should have a good understanding of these tools. It is not enough to just call like a ChatGPT API. That doesn’t help you because, in the competitive world, it’s not a competitive advantage. So to create competitive advantage if you want to do anything in AI/ML, one of the best ways of doing that is understanding these tools very in-depth.”